The classic on-premise data warehouse and its problems
Many companies use data warehouses (DWH) to store business data centrally in one place. This data store serves as the basis for complex analyses in the context of business intelligence (BI) and analytics. In a DWH, data from various heterogeneous data sources is extracted, harmonised and stored so that it can be used for analyses and evaluation, thereby supporting management decisions.
Setting up and operating a classic on-premise DWH involves high upfront investment costs. This is due on the one hand to the provision of large storage capacities, which are required to store large amounts of data, and on the other hand to high computing capacities, which require complex queries to be executed efficiently in an adequate amount of time. The ongoing operation of an on-premise DWH is also cost- and personnel-intensive due to resource management, monitoring and performance optimization, etc.
BigQuery as a cloud-based alternative to on-premise data warehousing
An alternative to an expensive on-premise Data Warehouse (DWH) is Google BigQuery. This is a cloud-based Enterprise Data Warehouse designed for advanced analytics within the Google Cloud Platform. BigQuery offers the capability to store data in the exabyte range and perform SQL queries in the petabyte range. Queries on datasets with millions of rows can be launched ad hoc and completed in a matter of seconds.
The core concept behind migrating or building a DWH in the cloud is the separation of storage and data processing (compute). This separation allows both components of a DWH to scale according to requirements and can be operated efficiently and cost-effectively. This flexible scaling is automatically driven by the volume of data and access demands, without the need for prior allocation of hardware resources. This is achievable because BigQuery is entirely serverless and can tap into all available resources of the Google Cloud Platform. As a result, unexpected workload spikes can also be accommodated, ensuring performance remains unaffected. This is why BigQuery avoids the typical over-provisioning seen in traditional DWH setups.
Due to its serverless architecture, BigQuery generally requires no planning or dimensioning of DWH configuration to meet performance requirements. The necessary storage and computing capacities are automatically utilised based on the volume of data.
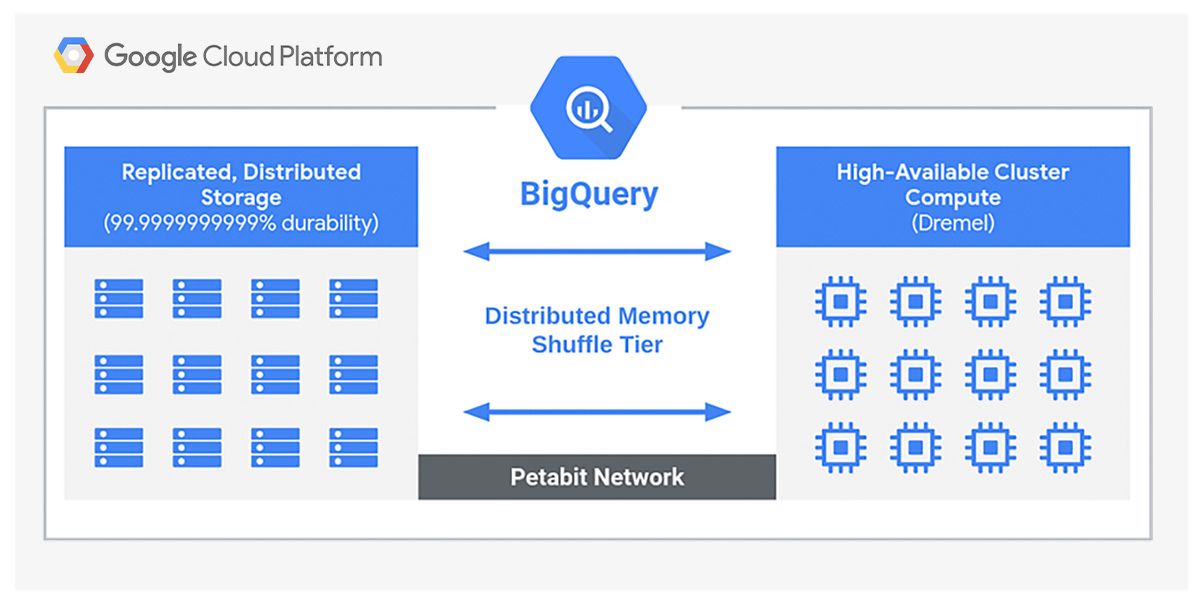
Cost advantages thanks to BigQuery
Unlike other cloud DWH solutions, BigQuery does not require contracts with minimum terms and upfront payments. The pay-as-you-go principle is used here. As with the other GCP services, only what is actually used has to be paid for. For companies that store and analyse large amounts of data, there is flat rate pricing, which further reduces costs. In total, BigQuery can save up to 52% of the total cost of ownership compared to an on-premise DWH. In addition, there is the option to automatically move data older than 90 days to low-cost storage to automatically reduce costs. Both cost management options offer maximum flexibility and customization for each customer.
As mentioned earlier, one of the biggest advantages of BigQuery is that there is no need for upfront investment in expensive DWH infrastructure. This makes BigQuery interesting for smaller and medium-sized companies where an investment in hardware is not worthwhile or possible. BigQuery offers the optimal opportunity to improve strategic decisions etc. with the help of data analytics.
Data security and high availability
BigQuery, like all GCP storage services, offers excellent resilience and high availability. By replicating data across multiple regions, it is protected from loss in the event of a data centre failure. At the same time, replication across multiple regions means that queries can continue to be executed in such a case, thus not restricting your own business processes.
The storage of all data is encrypted by default and meets all legal requirements for data protection and privacy. This also includes the free choice of the geographical region in which the data is stored by BigQuery. In addition, the data is protected from attacks and unauthorised writing/reading by Google's own security technologies. These technologies are constantly being developed further in order to be able to securely fend off the latest threats.
Prepared for the future with BigQuery
With BigQuery, companies can get real-time insights into business data and generate insights from massive amounts of data without the upfront hardware or software investments described above. Analytical capabilities range from ad-hoc analysis of instantaneous demand to dashboards for continuous monitoring of business activity, for example, using the Looker BI Platform. Such queries can be easily performed via the web browser-based UI or REST interface and exported as spreadsheets, for example.
Furthermore, BigQuery is the only cloud DWH solution that provides native support for artificial intelligence and machine learning. Both techniques are becoming increasingly important in the field of data analytics. The application of both technologies helps to generate more information for management decisions and thus to identify and fully exploit potential growth opportunities. BigQuery lays the foundation to benefit from both technologies in the best possible way.